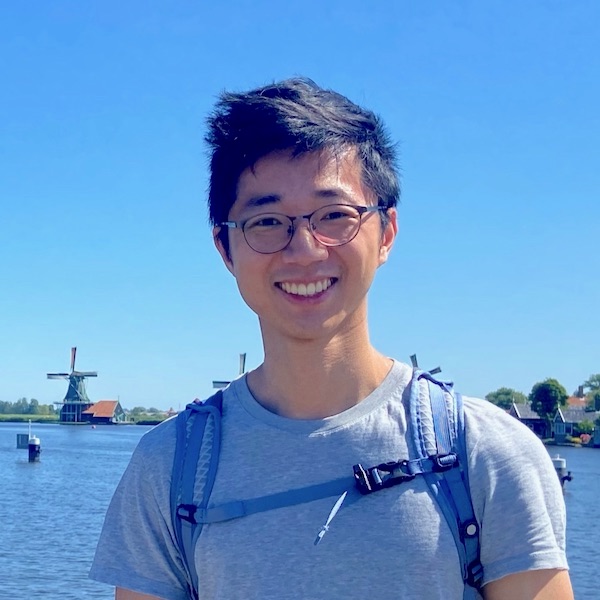
Alex L. Wang
Assistant Professor of Quantitative Methods
Daniels School of Business
Purdue University
Office: KRAN 463
Email: wang5984@purdue.edu
[G. Scholar] [arXiv] [OO]
If you are a motivated student interested in optimization and/or data science, I encourage you to apply to Purdue’s PhD program in Quantitative Methods and to mention my name in your application.
About
I am an assistant professor at Purdue University in the Daniels School of Business (Quantitative Methods Group).
I received my Ph.D. from the Computer Science Department at Carnegie Mellon University (2022), where I was supervised by Fatma Kılınç-Karzan. In Fall 2022, I was a postdoctoral researcher at Centrum Wiskunde & Informatica in the Optimization for and with Machine Learning project, where I was supervised by Monique Laurent.
My work has been recognized by the INFORMS Optimization Society 2021 Best Student Paper Award and an ICML Outstanding Paper Award 2022.
Research interests
My research focuses on extending classical optimization theory towards modern settings, especially those inspired by data science tasks. Recent topics of interest include: semidefinite programming, first-order methods, and nonconvex quadratic programming.
Recent papers:
- Accelerated Objective Gap and Gradient Norm Convergence for Gradient Descent via Long Steps
(ɑ) Benjamin Grimmer, Kevin Shu, and Alex L. Wang
March 2024
[arXiv] [mathematica]- This paper significantly shortens and strengthens the analysis for the stepsize sequences first described in our previous technical report Accelerated Gradient Descent via Long Steps.
-
Sharpness and well-conditioning of nonsmooth convex formulations in statistical signal recovery
(ɑ) Lijun Ding and Alex L. Wang
July 2023
[arXiv] [code] - Hidden convexity, optimization, and algorithms on rotation matrices
(ɑ) Akshay Ramachandran, Kevin Shu, and Alex L. Wang
Math. Oper. Res., to appear (2024)
[arXiv]